Subnet 15
De-Val
De-Val
De-Val is a decentralized subnet that evaluates LLM outputs for accuracy, completeness, and relevance.
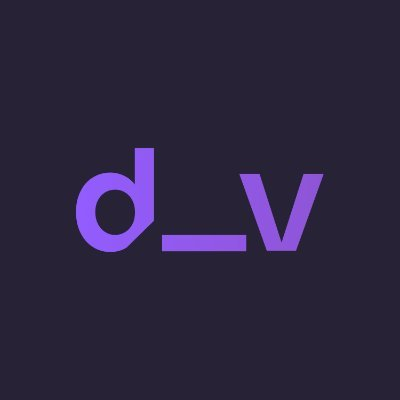
SN15 : De_Val
Subnet | Description | Category | Company |
---|---|---|---|
SN15 : De_Val | Inference verification & optimization | Generative Al | Manifold |
De-Val is a groundbreaking decentralized evaluation subnet for large language models (LLMs). Leveraging the robust Bittensor network, De-Val sets a new standard in evaluating LLM outputs with unparalleled objectivity and reliability. They address key challenges such as hallucinations, misattributions, relevancy, and summary completeness.
Thier innovative approach begins with using synthetic data for initial training. Looking ahead, they plan to offer comprehensive evaluation and monitoring capabilities for businesses aiming to refine their LLM-based solutions. This includes tools for A/B testing and offline validation. Specifically, they focus on evaluation within Retrieval-Augmented Generation (RAG) scenarios to help businesses answer critical questions such as:
- How accurate is my LLM given the provided context?
- How comprehensive are our abstractive summaries? Are we missing crucial details?
- Can we accurately identify key users and align their names with relevant action items?
In thier initial rollout, the subnet will feature evaluators dedicated to:
- Hallucination Detection: Spotting false or nonsensical outputs within LLM summaries in the context of RAG.
- Misattribution Correction: Assessing the accuracy of participant attribution in LLM summaries from sales calls, meetings, and general chat messages.
- Summary Completeness: Analyzing the thoroughness and reliability of generated summaries.
- Relevancy: Providing binary predictions on whether a response is pertinent to a user’s query.
Key Features
Unbiased, Decentralized Evaluations
Harnessing decentralization to ensure impartial and dependable assessments. Community-driven evaluations promote ongoing improvement.
Advanced Evaluation Metrics
Specialized evaluators for detecting hallucinations, correcting misattributions, and evaluating summary completeness. Regular updates based on community feedback and evolving requirements.
Cost-Effective Solutions for SMBs
Affordable access through flexible pay-per-query API or subscription models. Customized solutions to fit specific business needs.
Detailed Feedback and Analytics
In-depth scoring from 0 to 1, offering actionable insights for optimizing RAG pipelines. User-friendly dashboards for monitoring and analyzing performance metrics.
Seamless Integration and Scalability
Simple APIs for integrating with current systems. Scalable solutions designed to adapt to your business’s growth.
Miner and Validator Functions
Miner
Receives evaluation queries from the Validator, which include context and LLM responses.
Uses advanced machine learning models to analyze the queries for hallucination detection and relevance.
Returns detailed evaluation scores to the Validator for further review and action.
Validator
Gathers evaluation results from Miners, including scores and analyses.
Compares these results to benchmarks and real-world data to verify accuracy and reliability.
Records the validation outcomes for auditing and ongoing enhancement of the De-Val evaluation system.
Awaiting Data