Subnet 02
Omron
Inference Labs
Omron enhances Bittensor with a Proof-of-Inference system to verify and secure AI-generated data and intelligence
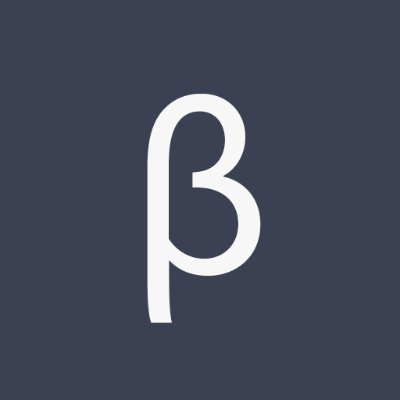
SN2 : Omron
Subnet | Description | Category | Company |
---|---|---|---|
SN2 : Omron | Inference verification (zkML) | Generative AI | Inference Labs |
Omron signifies a substantial advancement in fortifying the Bittensor network, with the goal of establishing the largest peer-to-peer Verified Intelligence network. This entails constructing a Proof-of-Inference system tailored for the Bittensor network. The Subnet focuses on using computation to provide authenticity guarantees for data or intelligence generated from models, ensuring that the source is as expected. Such an endeavour is in line with the Opentensor foundation’s standards for pioneering subnet solutions.
The goal is to guarantee that the data or intelligence received comes from the specific AI model intended, crucial for open-source models. This verification ensures that users can trust the origins of the generated intelligence, addressing concerns about data authenticity in AI applications.
Comprising a Bittensor subnet and an Ethereum deployment, Omron allows users to deposit Liquid Staking Tokens (LST) on Ethereum, receiving a restaking token and points in return. This restaking token, accompanied by rewards, grants users the freedom to utilize their assets across various EVM ecosystems. Moreover, it furnishes verified machine learning data, facilitating the development of cutting-edge multipurpose LST strategy models.|
It aims to optimize and verify liquidity staking and re-staking strategies through artificial intelligence and machine learning technologies. Omron uses smart contracts and verification nodes to provide automated re-staking strategies and ensures the authenticity and security of the reasoning process through a zero-knowledge proof mechanism.
Off-loading computational tasks off-chain prevents bloating the network, enabling easier verification of computations. Proof of inference systems facilitate verifying computations that happen off-chain, providing evidence of the process without burdening the network. Proof of inference plays a crucial role in ensuring the authenticity of AI models running subnets, allowing for secure allocation of user funds.
Functionality of Miners and Validators
Omron introduces incentives for miners and validators within Subnet 2, encouraging their involvement in generating and validating high-quality, secure, and efficient AI predictions. This specialized reward system is tailored to the unique features of zero-knowledge machine learning (zk-ML) and decentralized AI. While zero-knowledge proofs are currently more CPU computationally intensive, allowing non-GPU miners to participate, the ultimate aim is to promote the development of proving systems optimized for GPU-based operations. Incentives are centred around miners crafting concise and efficient models, which can be circuited using a zero-knowledge proving system.
The reward mechanism for Subnet 2 evaluates initial AI predictions based on cryptographic integrity and the time taken to generate zk-proofs, in addition to the outputs, rather than solely focusing on end results. This methodology alleviates the computational load on validators, as zk-proofs efficiently confirm the source model and the integrity of AI predictions.
Miners:
- Receive input data from validators within the subnet.
- Utilize custom, verifiable AI models, converted into zero-knowledge circuits, to generate predictions.
- Return the generated content to the requesting validator for validation and distribution.
Validators:
Evaluate results from miners based on performance metrics such as proof size and response time.
Generate input data and distribute requests for verified inference among participating miners within the subnet.
Verify the authenticity of miners’ returned zero knowledge proofs to ensure faithful action.
The team have experience in civil aviation AI projects and social AI experiments which led up to their involvement with Bittensor. Delving into questions about AI model origins and royalties distribution, the team recognized the potential of blockchain for ensuring authenticity and fair compensation in AI collaborations. By identifying the need for proof of inference in the AI space, Inference Labs found a specific problem to solve with broad applications, including across web 2 and web 3 platforms.
Colin Gagich – Co-Founder
Ronald Chan – Co-Founder
Spencer Graham – Software Developer
Will P – Software Developer
Ehsan Meamari – Researcher
Julia Théberge – Executive Assistant
Shawn Knapczyk – Communities Manager
Ivan Anishchuk – Crypto Researcher
Jonathan Gold – Software Engineer