Subnet 21
Any-to-Any
Omega Labs
The Any-to-Any Subnet pioneers decentralized multimodal AI models, leveraging vast datasets and global research
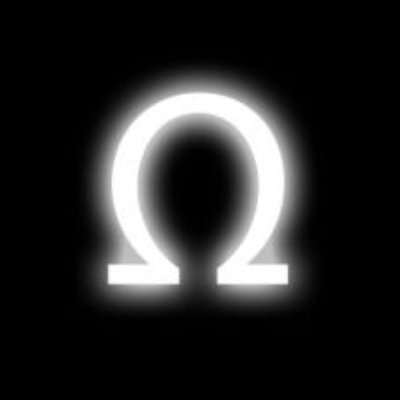
SN21: Omega Any-to-Any
Subnet | Description | Category | Company |
---|---|---|---|
SN21: Omega Any-to-Any | Multimodal models | Model development | Omega Labs |
The Any-to-Any Subnet, developed by OMEGA Labs on the Bittensor blockchain, represents a decentralized, open-source AI initiative. Their mission is to pioneer state-of-the-art multimodal any-to-any models, drawing in top AI researchers globally to leverage Bittensor’s incentivized intelligence platform for training and compute contributions. Their vision includes establishing a self-sustaining research lab where participants are rewarded for advancing AI capabilities through computing resources and research insights.
Boasting a dataset that includes over 1 million hours of footage, 30 million video clips, and expanding coverage of 50+ scenarios and 15,000+ action phrases. This dataset places them as a significant contender in AI training datasets, challenging the scale of even prominent collections like YouTube’s 8 million video IDs. This initiative signifies a major advancement in decentralized AI on the Bittensor network, powered by high-quality data hosted on Huggingface, solidifying their position at the forefront of AI research and development
Subnet 21 is poised to redefine AI training with the world’s largest multimodal dataset. Over 30 million videos are fueling advanced models, empowered by $TAO. By leveraging the decentralized Bittensor network, Omega Labs stands at the forefront of revolutionizing open AGI research. They provide an unparalleled scale of multimodal data, addressing challenges such as the ARC-AGI benchmark. Their goal is to surpass traditional LLMs and closed-source initiatives, fostering pioneering open-source innovation.
Multimodal Approach: A2A integrates all modalities (text, image, audio, video) concurrently, driven by their belief that true intelligence emerges from associative representations at the intersection of these modalities.
Unified Representation of Reality: The Platonic Representation Hypothesis suggests that as AI models scale, they converge towards a fundamental representation of reality. A2A models, by jointly modeling all modalities, allow them to capture this structure, potentially accelerating progress towards more generalized AI.
Decentralized Data Collection: Through their SN24 data collection, they leverage a continuous flow of data mirroring real-world demand distribution for training and evaluation. Refreshing topics based on data gaps helps them mitigate underrepresented data classes, ensuring robust training via self-play among their subnet’s top checkpoints.
Incentivized Research: With Bittensor’s model for incentivizing intelligence, world-class AI researchers and engineers can be permissionlessly compensated for their efforts and have their compute subsidized according to their productivity, which they believe fosters open-source innovation.
Subnet Orchestrator: Their Bittensor Subnet Orchestrator integrates specialized models from other subnets, serving as a high-bandwidth router. As the leading open-source multimodal model, their platform enables future AI projects to bootstrap their expert models using rich multimodal embeddings.
Public-Driven Capability Expansion: They prioritize learning capabilities based on public demand through decentralized incentives.
Beyond Transformers: They integrate cutting-edge architectures like early fusion transformers, diffusion transformers, liquid neural networks, and KANs to expand their model’s capabilities beyond traditional transformer frameworks.
awaiting data