Subnet 24
Omega
OMEGA Labs
OMEGA Labs subnet is building the world’s largest decentralized multimodal dataset to advance AGI research
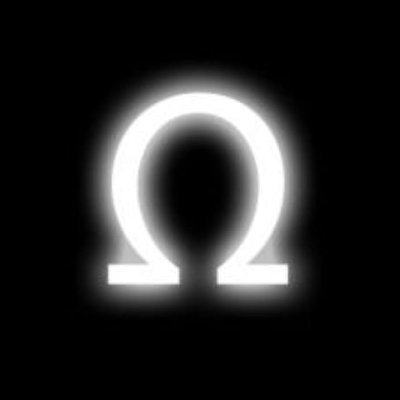
SN24 : Omega Labs
Subnet | Description | Category | Company |
---|---|---|---|
SN24 : Omega Labs | Multimodal datasets | Data Pipeline | Omega Labs |