Subnet 37
Finetuning
Macrocosmos & Taoverse
The Finetuning Subnet focuses on efficient AI model fine-tuning for improved performance
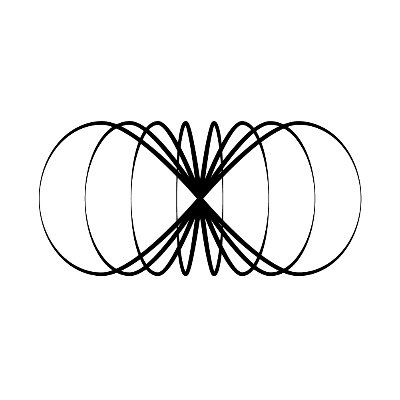
SN37 : Fine-tuning
Subnet | Description | Category | Company |
---|---|---|---|
SN37 : Fine-tuning | LLM fine-tuning | Decentralized Training Model development | Macrocosmos |
Refining machine learning models through fine-tuning plays a pivotal role in meeting user needs. This process, which demands significant time and computational resources, transforms raw outputs into actionable intelligence, marking the final phase of AI model development.
That’s why Taoverse has launching Subnet 37, a new fine-tuning subnet, in partnership with Macrocosmos. Leveraging Taoverse’s engineering expertise and Macrocosmos’ deep AI talent and subnet design proficiency, Building on the work of Nous Research their collaboration aims to set a new benchmark for fine-tuning within the Bittensor ecosystem. This initiative has the potential to enhance performance across the entire network.
Nous Research’s AI team have demonstrated significant demand for a fine-tuning subnet, underscoring its critical role in advancing the AI ecosystem on Bittensor. As Macrocosmos expand their subnet portfolio to support broader AI goals within the Bittensor community, efficient fine-tuning of models becomes increasingly vital. Building upon the foundation laid by Nous Research and leveraging synergies with Macrocosmos’ subnets like SN9, SN1, and SN13, Taoverse aims to bring fine-tuning capabilities to Bittensor.
Subnet 37 rewards miners for fine-tuning Large Language Models (LLMs). Initially, the inaugural competition assesses performance using a continuous flow of synthetic data sourced from subnet 18, ensuring a dynamic fine-tuning benchmark updated daily.
The technical requirements for operating a fine-tuning subnet are substantial. It necessitates integrating the appropriate pre-trained base models, datasets, evaluation mechanisms, and ample computational resources, all while aligning with the decentralized nature of the Bittensor ecosystem. What may suffice in a closed, centralized system may fall short in an open, competitive environment like Bittensor.
By combining Taoverse’s engineering prowess with Macrocosmos’ operational experience and community support, they are poised to deliver the benefits of fine-tuning to Bittensor. With Subnet 37, they are integrating the entire AI production line into Bittensor: leveraging SN9’s pre-trained models for fine-tuning, thereby supporting their goal of training models for inference across the ecosystem. This holistic approach enables them to realize the potential of a highly efficient ML system, where insights from SN13 and SN1 are pre-trained in SN9, fine-tuned in SN37, and then integrated back into SN1.
However, achieving this vision requires ongoing collaboration with their community. Intelligence is intricate, and training it on distributed networks is even more challenging. Success hinges on continuous community support, ensuring that fine-tuning on Bittensor reaches its full potential and enhances overall ecosystem performance.
Incentive Mechanism
Within this subnet, miners are evaluated based on how often the models they host achieve lower losses compared to other models on the network during competitions. To succeed, miners must achieve the lowest loss across a significant number of random batches. Early identification of the best model and delta in each competition maximizes incentives.
It’s important to note that competitions are independently specified within this subnet, with predefined allocations of emissions. Each competition has unique parameters defining the models, tokenizers, sizes, sequence lengths, and other criteria against which miners will be evaluated.
Will Squires – CEO and Co-Founder
Will has dedicated his career to navigating complexity, spanning from designing and constructing significant infrastructure to spearheading the establishment of an AI accelerator. With a background in engineering, he made notable contributions to transport projects such as Crossrail and HS2. Will’s expertise led to an invitation to serve on the Mayor of London’s infrastructure advisory panel and to lecture at UCL’s Centre for Advanced Spatial Analysis (CASA). He was appointed by AtkinsRéalis to develop an AI accelerator, which expanded to encompass over 60 staff members globally. At XYZ Reality, a company specializing in augmented reality headsets, Will played a pivotal role in product and software development, focusing on holographic technology. Since 2023, Will has provided advisory services for the Opentensor Foundation, contributing to the launch of Revolution.
Steffen Cruz – CTO and Co-Founder
Steffen earned his PhD in subatomic physics from the University of British Columbia, Canada, focusing on developing software to enhance the detection of extremely rare events (10^-7). His groundbreaking research contributed to the identification of novel exotic states of nuclear matter and has been published in prestigious scientific journals. As the founding engineer of SolidState AI, he pioneered innovative techniques for physics-informed machine learning (PIML). Steffen was subsequently appointed as the Chief Technology Officer of the Opentensor Foundation, where he played a pivotal role as a core developer of Subnet 1, the foundation’s flagship subnet. In this capacity, he enhanced the adoption and accessibility of Bittensor by authoring technical documentation, tutorials, and collaborating on the development of the subnet template.
Pedro Ferreira – Machine Learning Engineer
Kalei Brady – Data Scientist
Sergio Champoux – Data Scientist
Brian McCrindle – Machine Learning Researcher
Elena Nesterova – Lead Technical Program Manager
Richard Hudson – Communications Lead
Alex Williams – Recruitment Lead