Subnet 47
Condense AI
Neural Condense
A decentralized subnet condensing lengthy contexts for efficient large language model processing and inference
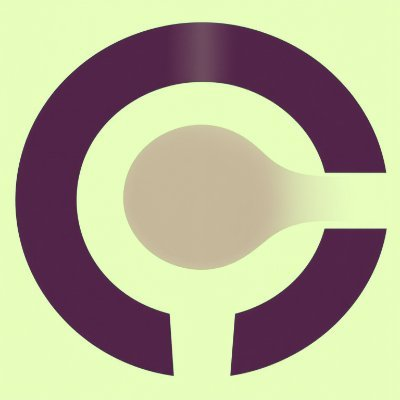
SN47 : Condenses AI
Subnet | Description | Category | Company |
---|---|---|---|
SN47 : Condenses AI | Token compression to reduce LLM inference costs | Model development | Condenses Al |
The computational demands of large language models (LLMs), particularly transformer-based architectures, grow significantly as input lengths increase due to the quadratic complexity of their self-attention mechanisms. This creates challenges for applications requiring extended context, such as Retrieval-Augmented Generation (RAG) and long-form conversational models.
The Condense AI subnet tackles this issue by employing miners to condense lengthy contexts into compact, meaningful token representations. By distributing the condensation process across a network of miners, the subnet efficiently manages large inputs, enabling LLMs to handle extended contexts with a reduced computational burden. The approach includes a robust architecture, an incentivized system for miners and validators, and offers transformative potential for a range of applications.
Their decentralized subnet for token condensation tackles the challenge of processing long sequences in large language models by significantly reducing computational complexity. This innovative approach boosts efficiency while fostering collaboration, with miners and validators driving the advancement of token compression techniques. Their vision is clear: to build a globally trusted decentralized network that accelerates AI inference, delivering cutting-edge performance at an accessible cost for engineers everywhere.
In lamens terms, it condenses LLM prompts into just the tokens needed to maintain context enabling faster and more cost-efficient prompting, and does so whilst maintaining privacy.
The Condense AI subnet tackles the challenges of long-context processing in large language models (LLMs) by condensing lengthy inputs into compact, meaningful token representations.
Key Features of the Subnet
Decentralized Token Condensation
They utilize a distributed network of miners to process and condense lengthy contexts into minimal token sets, preserving essential information while reducing computational loads. This parallelized approach accelerates processing and optimizes tasks such as Retrieval-Augmented Generation (RAG) and conversational models.
Incentivized Ecosystem
Validators assess miners’ performance using synthetic challenges that simulate real-world scenarios. These tasks ensure miners effectively condense data while maintaining context integrity. Miners are incentivized through tiered rewards, encouraging consistent performance and innovation.
Efficient API for LLM Engineers
Their subnet API empowers engineers to optimize long-context applications by condensing thousands of tokens into compact “soft tokens” that retain context while reducing computational demands. This results in faster and more cost-efficient LLM inference.
Support for Advanced Models
The subnet is designed to evolve, initially adding new models to a research tier for miner experimentation before advancing them to an inference tier for production use. This ensures seamless scaling and optimization as new technologies emerge.
Miner and Validator Operations
Validators:
- Validators set rate limits, issue challenges, benchmark miners’ performance, and score their outputs. They play a pivotal role in maintaining the system’s reliability and accuracy.
Miners:
- Miners refine their compression algorithms, adapt to evolving tiers, and compete to achieve high validation scores. They are encouraged to collaborate and innovate, supported by educational resources and community events.
Benefits and Commercialization
Accelerated Inference
By reducing input sizes, they enable faster processing and lower computational costs, catering to engineers of RAG systems, chat frameworks, and LLM optimization projects.
Flexibility for Model Support
The subnet is adaptable to various models and workflows, ensuring its applicability to diverse industries.
Community-Driven Innovation
Through active research collaboration and incentivized performance, the subnet fosters an ecosystem where token compression techniques continually improve.
Their subnet is transforming long-context processing in LLMs, providing engineers with efficient, scalable, and decentralized solutions for advanced applications.
Awaiting Data